Bridging Innovation and Inspiration
July 1, 2025This is the 1st article in a 3-part series written by ETH Students from the ETH Studio Heilbronn.
My name is Giancarla Salzarulo and I am from Italy. I recently completed my Master’s thesis in computational immunology as an international student at ETH Zurich. I worked in the Reddy Group at the Department of Biosystems Science and Engineering (D-BSSE) in Basel, a lab specializing in systems and synthetic immunology, integrating genomics, molecular biotechnology and immunology. My thesis aimed to quantitatively understand immune responses using experimental and computational methods, an experience that deeply shaped my academic path.
Currently, I’m still in Basel, finishing an internship at Roche and exploring my next career steps at the intersection of biotech, data science, and healthcare innovation. This is why I was deeply honoured to be selected as part of the ETH Zurich alumni delegation to attend the TECH Conference 2025 in Heilbronn. With over a thousand innovators from academia, industry and policy present, the conference provided a dynamic platform to explore and discuss Europe’s technological future and ETH Zurich had a strong and visible presence throughout.
It was especially exciting to see ETH expanding beyond the Swiss borders with the planned Heilbronn campus. I was proud to be part of a community that continues to grow globally. Meeting members of the ETH delegation reminded me that ETH is a vibrant network of passionate thinkers and doers. The talks were just as impactful as the people I met. I was particularly moved by the session from Bill Anderson, CEO of Bayer. Thanks to a tip from my manager, I learned he was previously CEO of Roche Pharmaceuticals, which made his message even more meaningful. He spoke about the urgent need to accelerate drug discovery through innovation. With my background in bioinformatics, I immediately saw the connections to my work at ETH and the broader mission of biotech companies. The challenges he described felt tangible and relevant.
Equally compelling was the keynote by Maria Ressa, Nobel Peace Prize laureate—a fearless advocate for press freedom and ethical technology. Her reflections on technology never being neutral struck a deep chord. In a world where innovation often outpaces ethics, her humanity and clarity were humbling and inspiring.
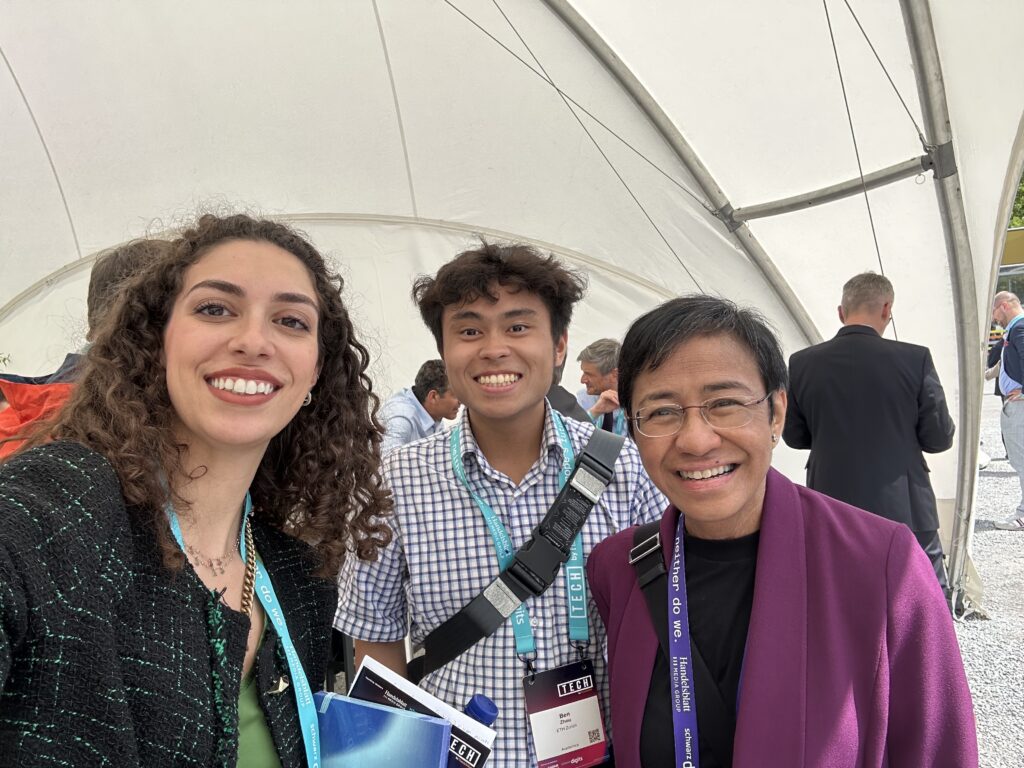
The conference took place across blue and purple stages and two spaces: the House of Learning, where many sessions were held, and the TECH forum showcasing emerging technologies. There, I had the unexpected opportunity to experience a virtual twin of my own heart. In a demo presented by Dassault Systèmes, I saw how simulation technology can transform medicine.
A highlight was the session by Lea Steinacker and Miriam Meckel, co-founders of Ada Learning, who explored digital sovereignty, a topic increasingly central to Europe’s technological identity.
Beyond the talks, the people I met made this experience truly memorable. I connected with brilliant individuals such as Michele De Lorenzi, Jürg Brunnschweiler, Boris Zürcher, Gisbert Schneider, Viktoria Österdahl, Annegret Kern, Sven Corus and more. I’m sincerely grateful for the engaging conversations and meaningful connections we created—moments that reminded me once again of the depth and reach of the ETH community.
More than a professional experience, TECH Conference 2025 became a personal turning point. As I approach the end of my internship at Roche and actively seek my next opportunity, the conference gave me not only motivation but also clarity. It helped me reflect on where I want to go, how I want to contribute and, perhaps most importantly, who I want to become.
I arrived with curiosity and left with inspiration. Being part of ETH Zurich’s story continues to be one of the greatest privileges of my life.
For additional details about the ETH delegation at the TECH Conference 2025, see this post.